Data strategy is the backbone of organisational data practices and a long term execution of a vision to gain an optimal performance around your enterprise data. It’s about taking iterative actions to inspect and adapt organisation wide data-driven operations. Additionally, it’s a long-term plan that characterises the people, processes, and technology required to resolve your data challenges and help accomplishing your key business objectives. In this article, we will get a bird-view of few of the key essentials required for a successful data strategy implementation.
Getting aligned with Organisation’s Vision & Business Strategy
To be truly valuable, data related capabilities should address specific business objectives. The result of any misalignment with business stakeholders could result in you run into the risk of prioritising the incorrect tasks, missing interesting KPIs, wasting time and resources, and even worst, losing the organisation’s interest and confidence in such larger data capabilities related initiatives.
A better, tangible and more successful outcome can be achieved if you are aligned with the business’s vision and strategy.
The entire organisation benefits when your data related projects contribute to realistic and valuable objectives. And that indirectly maximises your gains on business goals, objectives, which increases the priority given to data operations.
Implementation of Data Strategy & Management Practices
There is a high chance to become a victim of being a data alien which results into waste of time, resources and most importantly focus and humanly energy. As a leader in data strategy domain, you must ensure the key artefacts such as Data Assets, Data Sources and Architectures and properly defined and portraying the real illustration of the organisation, this would help counter upcoming challenges.
Data Assets:
Data assets refer to the data of any form. Artefacts, such as files, databases, diagrams, spreadsheets, etc. Listing and defining all the related data assets could be the first step.
Benefits:
- Assesses data landscape rationally
- Helps connecting all the dots around data
- Improves data transparency
- Unlocks business value
Data Catalog:
Another way of collection of data assets could be using Data Catalog. Data catalog tools could be very helpful in automising the whole metadata and data assets collection from existing business data.
Benefits:
- Improves data search and discovery
- Saves time and resources over manual data assets collection
- Amplifies operational efficiency
- Consolidates metadata from multiple data sources
- Streamlines metadata collection by automation
Data Sources:
Generally data source is defined as the original location of data where it was born or stored for the first time. For instance, a database, a log server, an SFTP file server, etc. Visually define all the data sources (both internal and external), so they make sense.
Benefits:
- Demystifies data movement
- Narrows down data search and catalog capabilities
- Helps having a control over data
- Avoids stacking up of unnecessary data
- Builds better integration layers
Data Lineage:
Data lineage defines the process of tracking data changes and where each data asset originates. Implementation of available tools can be very beneficial but one has to be extra cautious as this might drive into requirements of extra resources.
Benefits:
- Advocates regulatory compliance
- Establishes trust among sources and reporting of data
- Contributes in improving Data Quality
- Highlights any impacts prior to their existence
Data Architectures:
You can also use our Pre-requisites Guide for designing the right Big Data architecture!
Data Architecture is defined as a blueprint of collection of data, it’s transformation, distribution, and consumption within an organisation. Mostly, it’s Data or Solutions Architects who are visually implementing data architectures related initiatives. A good practice is to reuse such illustrations with the support of SAs or DAs.
Benefits:
- Establishes vision-driven data ecosystem
- Maximises business value
- Helps aligning all the stakeholders
- Showcases a bird-view of data asset flow
- Encourages having data as a Single-Source-Of-Truth
- Identifies data duplications
- Applies and enforces best practices
Data Governance & Data Quality:
By definition, Data Governance is a set of certain principles and practices that ensure high quality, availability, modelling and security of data through the complete lifecycle of your data, defined by roles. It also helps defining a framework resulting in actionable insights for stakeholders for larger initiatives and decisions. A properly defined framework helps getting aligned within and cross departmental value streams.
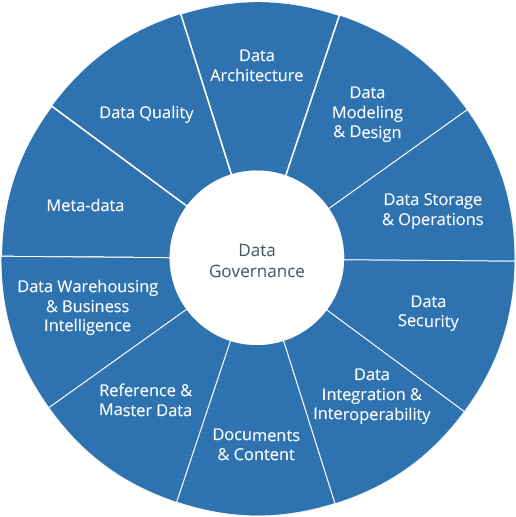
Ref: https://www.dama.org/sites/default/files/download/DAMA-DMBOK2-Framework-V2-20140317-FINAL.pdf
Benefits:
- Enables data trustworthiness
- Minimizes data inconsistencies
- Helps aligning business and regulatory requirements
- Helps avoidance of data-in-silos and data swamps
- Defines roles and responsibilities
- Identifies any obstacles
- Helps taking prior actions to resolve those obstacles
- Delivers high quality data
NOTE:
Ensure all such documentation is centrally available for all the stakeholders.